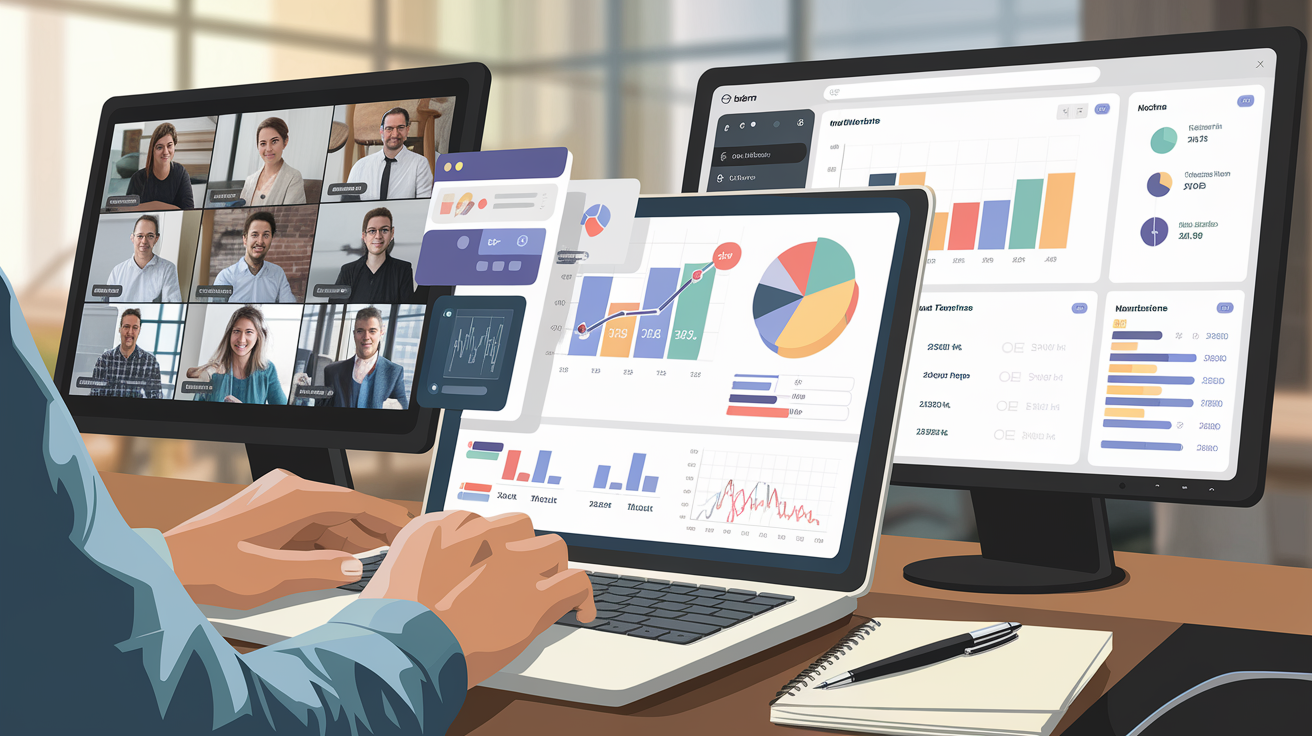
Predictive analytics is reshaping digital marketing by giving businesses the tools to anticipate customer behaviors, optimize campaigns, and maximize ROI. Powered by AI and machine learning, predictive analytics turns raw data into actionable insights, enabling marketers to create more targeted, efficient, and impactful strategies.
Let’s explore the growing role of predictive analytics in 2024 and how businesses can use it to stay ahead of the competition.
What is Predictive Analytics?
Predictive analytics involves analyzing historical data to forecast future outcomes. By using advanced algorithms and AI, businesses can predict trends, customer behaviors, and campaign performance.
Key components of predictive analytics include:
• Data Collection: Gathering data from multiple sources, such as website interactions, social media, CRM systems, and sales data.
• Machine Learning Models: Training algorithms to recognize patterns and make predictions.
• Actionable Insights: Turning predictions into practical recommendations to guide marketing strategies.
In essence, predictive analytics helps marketers move from reactive strategies to proactive decision-making.
1. Enhanced Audience Targeting
One of the most significant benefits of predictive analytics is its ability to identify and target the right audience.
• Segment Analysis: AI identifies audience segments most likely to engage or convert, based on past interactions and behaviors.
• Lookalike Audiences: Predictive tools find potential customers who resemble your most valuable audience, helping expand your reach effectively.
• Churn Prediction: Predictive models can identify customers at risk of leaving and trigger retention campaigns to re-engage them.
Example: An e-commerce platform can use predictive analytics to target users who are likely to abandon their shopping carts with personalized offers, reducing lost sales.
Why it matters: Focusing on high-probability prospects ensures efficient use of marketing budgets and higher conversion rates.
2. Optimizing Campaign Performance in Real Time
Predictive analytics doesn’t just forecast outcomes—it enables marketers to adjust campaigns on the fly.
• Real-Time Insights: AI tracks campaign performance in real time and identifies areas for improvement, such as poorly performing ads or under-targeted segments.
• Budget Allocation: Predictive tools recommend reallocating budgets to high-performing channels or campaigns.
• A/B Testing: Predictive analytics identifies which creative elements (e.g., headlines, images, or CTAs) are most likely to succeed based on historical performance.
Example: A fashion brand can dynamically shift ad spend from underperforming display ads to more effective social media campaigns during a holiday sale.
Why it matters: Real-time optimization minimizes wasted spend and ensures every marketing dollar works harder.
3. Sales and Demand Forecasting
Predictive analytics provides insights that go beyond marketing, helping businesses anticipate demand and align sales efforts with customer needs.
• Seasonal Trends: AI identifies periods of high demand and recommends the best times to launch promotions or campaigns.
• Inventory Planning: Predictive models forecast which products are likely to sell well, ensuring businesses stock accordingly and avoid shortages or overstocking.
• Sales Pipeline Analysis: Predictive tools assess the likelihood of closing deals and prioritize high-potential leads.
Example: A SaaS company uses predictive analytics to determine which prospects are most likely to convert during a free trial and focuses its sales efforts on these accounts.
Why it matters: Accurate forecasting leads to better resource allocation, fewer missed opportunities, and smoother operations.
4. Customer Lifetime Value (CLV) Predictions
Understanding the long-term value of customers is critical for creating sustainable marketing strategies. Predictive analytics helps businesses estimate CLV and allocate resources accordingly.
• High-Value Customers: Identify customers who are likely to bring the most revenue over time and invest in retention strategies.
• Loyalty Programs: Predict which incentives are most effective for boosting repeat purchases among valuable customers.
• Upselling and Cross-Selling: Forecast which products or services a customer is likely to purchase next and tailor recommendations accordingly.
Example: A subscription-based business can predict when customers are likely to churn and offer incentives like discounts or exclusive benefits to retain them.
Why it matters: CLV predictions enable businesses to focus on long-term growth instead of short-term gains.
5. Predicting Content and Channel Effectiveness
Predictive analytics can also guide content strategies by identifying which types of content resonate most with specific audience segments.
• Content Recommendations: AI analyzes past engagement data to suggest blog topics, video formats, or social media trends that are likely to perform well.
• Channel Insights: Predictive models determine which platforms (e.g., Instagram, LinkedIn, or email) will yield the highest engagement for a given campaign.
• Timing Optimization: AI forecasts the best times to post content or send emails for maximum impact.
Example: A B2B company uses predictive analytics to decide whether to focus more on LinkedIn webinars or email newsletters based on past attendance and open rates.
Why it matters: Aligning content and channel strategies with audience preferences drives better engagement and ROI.
6. Fraud Detection and Risk Management
In addition to improving marketing outcomes, predictive analytics plays a vital role in protecting businesses from fraud.
• Ad Fraud Prevention: AI detects fraudulent clicks or impressions, ensuring ad spend isn’t wasted.
• Payment Risk Assessment: Predictive models identify suspicious transactions, reducing chargebacks and losses.
• Brand Safety: AI monitors ad placements to prevent associations with inappropriate or harmful content.
Example: An ad agency uses predictive analytics to flag unusual traffic patterns, protecting its clients from click fraud.
Why it matters: Fraud prevention ensures marketing resources are used effectively and protects brand reputation.
Challenges of Predictive Analytics
While predictive analytics offers immense value, businesses must navigate challenges to use it effectively:
1. Data Quality: Accurate predictions require high-quality, comprehensive, and up-to-date data. Gaps or inconsistencies in data can skew results.
2. Complexity: Implementing predictive models often requires technical expertise and the right tools.
3. Privacy Concerns: Collecting and analyzing user data must comply with regulations like GDPR and CCPA. Transparency with customers is essential.
Key Tools for Predictive Analytics
Businesses looking to leverage predictive analytics can explore tools like:
• Google Analytics 4 (GA4): Advanced predictive insights for web and app traffic.
• HubSpot: Built-in AI-powered forecasting tools for marketing and sales.
• Salesforce Einstein: Predictive tools tailored for CRM and sales teams.
• Tableau: Data visualization software for exploring predictive insights.
• Microsoft Power BI: Combines predictive analytics with data reporting capabilities.
How Predictive Analytics Benefits Businesses
1. Proactive Decision-Making: Marketers can anticipate challenges and opportunities before they arise.
2. Higher ROI: Resources are allocated more efficiently, driving better outcomes with lower costs.
3. Improved Customer Experiences: Personalization based on predictive insights creates more meaningful and timely interactions.
Predictive analytics is a game-changer for businesses in 2024, enabling smarter campaigns and better decision-making. By leveraging the power of AI and data, companies can move beyond guesswork to deliver targeted, impactful, and cost-effective marketing strategies.
Want to integrate predictive analytics into your marketing efforts? Contact appex.media to learn how our AI-driven solutions can help you unlock your data’s full potential.
Comments are closed